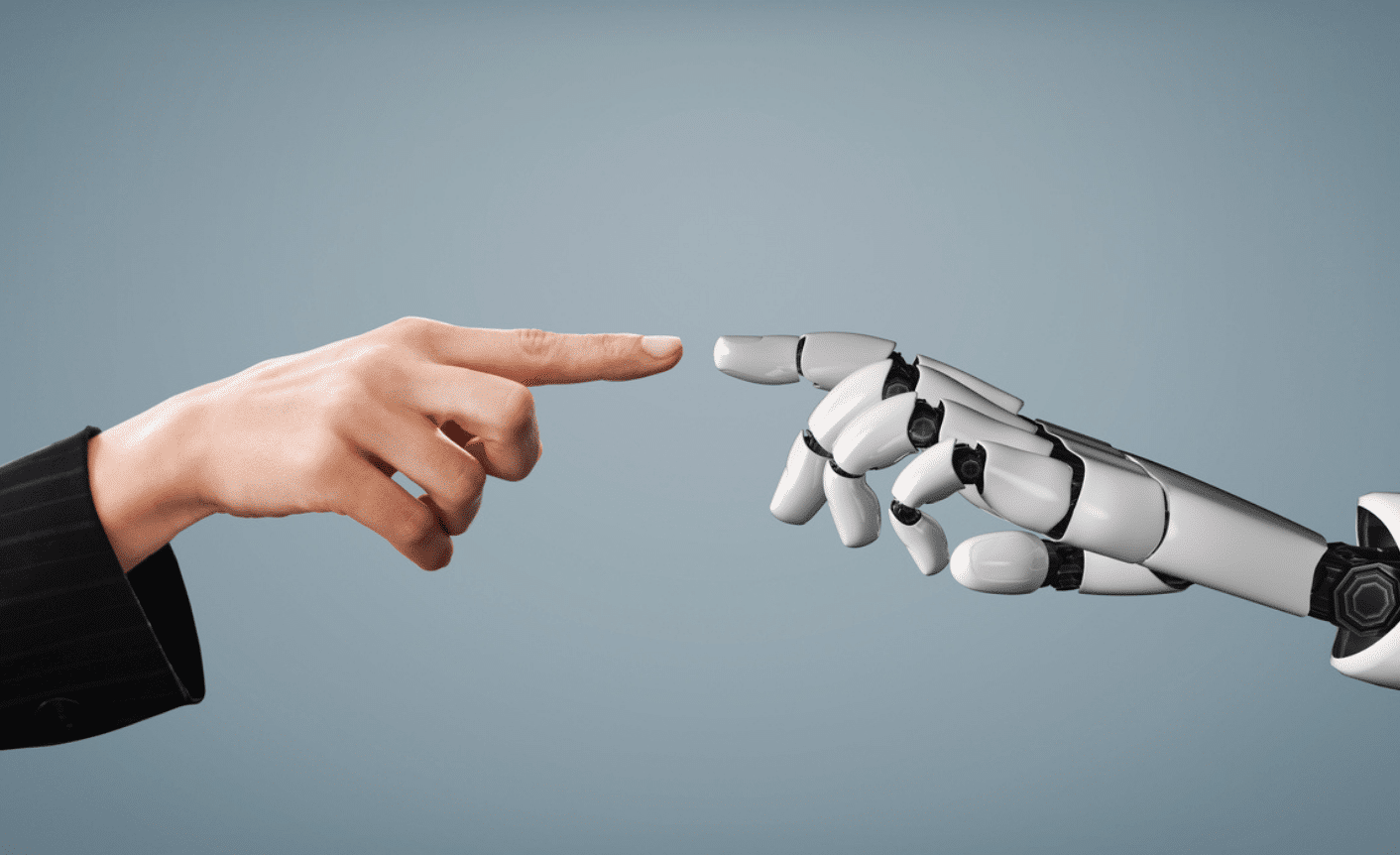
Most of us don’t spend much time thinking about how ingrained technology is with our ability to make decisions. It assists us in almost every facet of life, but the truth is — it’s not fair to everyone. Unfortunately, there’s no shortage of algorithmic bias examples that prove technology is only as inclusive as the researchers and data set it’s build by. This blog explores the role of technology in diversity and inclusion, by unpacking algorithmic bias.
Artificial Intelligence helps people make decisions in the medical field, self-driving vehicles, facial recognition software, chatbots, and recruitment processes. It’s also used in courts to predict the probability of a person recommitting a crime, just to name a few. Sometimes, we let technology make decisions for us entirely, dangerously assuming that it’s more objective than human judgment.
History in itself contains bias because it doesn’t record everyone’s perspective. And history has greatly influenced modern algorithms because we generated massive data sets that consist of decades of information built on exclusion and discrimination. When researchers design modern algorithms that rely on these databases to make automated decisions, it results in algorithmic redlining. That means algorithms are directly reproducing, reinforcing, and perpetuating pre-existing segregation and bias (Allen, J. 2019)
But does it have to be that way? Is it possible to use algorithms to advance our diversity and inclusion efforts? Or is it simply impossible to create fair and inclusive artificial intelligence?
What is algorithmic bias?
It makes sense that AI can either be as discriminatory or inclusive as the people designing and developing it. But unless you’re someone who is directly affected by AI bias, it might not seem as obvious to you. For someone like Joy Buolamwini, it was impossible to ignore.
MIT grad student and researcher Joy Buolamwini uses art and research to illuminate the social implications of Artificial Intelligence. Her work has led her to illuminate the need for algorithmic justice at the World Economic Forum and the United Nations. She now serves on the Global Tech Panel to advise world leaders and technology executives on ways to reduce the harms of AI. Her journey started when she was working with facial analysis software — that couldn’t detect her face unless she put on a white mask. Why? Because the algorithm was biased. This bias happened because the people who coded the application didn’t use a diverse data set. That meant the algorithm couldn’t identify a diverse range of skin tones and facial structures. And when it could, it often paired the wrong gender to African American faces.
How do algorithms become unfair?
Let’s make one thing clear, algorithms themselves aren’t inherently biased. They’re just mathematical functions: a list of instructions designed to accomplish a task or solve a problem. It’s the types of data that train the machine learning model, and who trains it, that introduce bias.
Data can be biased for different reasons. It could be due to:
- Lack of minority representation.
- Model features that are associated with race/gender. For example, due to the history of racial segregation in South Africa, where you live can predict your skin tone.
- The training data itself reflects historical injustice, which is then captured in machine learning models. (O’Sullivan, 2021)
Algorithmic bias is a systematic error (a mistake that’s not caused by chance) that causes unfair, inaccurate, or unethical outcomes for certain individuals or groups of people. Left unchecked, it can repeat and amplify inequality and discrimination and undermine our diversity and inclusion efforts. Thankfully, ethical practices are slowly emerging for developing more diverse and inclusive algorithms.
Want to know more about algorithmic bias? There’s a recent documentary called “Coded Bias,” that features the work of Joy Buolamwini. It’s a must-watch for anyone who uses search engines or artificial intelligence in their daily lives.
Is inclusive and ethical AI possible?
Put simply, technology must play a role in our diversity and inclusion efforts. Why? Because the average person spends 3 hours and 15 minutes a day looking at a screen. Everything we read, watch, listen to, and interact with in the digital world influences the way we perceive, think, work, dress, parent, talk, and move in the physical world. There’s an incredible incentive there to make digital spaces inclusive and accessible for everyone. A good starting point might be to stem the spread of biased or inaccurate information (and data). Diversity simplify describes the various ways to be human, and researchers who use diverse data sets increase the accuracy of the technology they’re developing to identify and predict outcomes for as much of humanity as possible.
Inclusive technology can only come from companies that cultivate an inclusive workplace culture. The good news is that “the innovative nature of tech companies allows them to push the limits of what organizations can do in terms of diversity and inclusion.” (Frost, Alidina, 2019).
Developing inclusive technology requires us to:
- Determine a clear definition of what fair and inclusive technology is.
- Seek diverse data sets. If the available data sets you’re using to develop your product aren’t diverse enough, consider involving external user experience research in the development process.
- Create a bias impact statement. A bias impact statement is a self-regulatory practice that can help prevent potential biases. It’s a “brainstorm of a core set of initial assumptions about the algorithm’s purpose prior to its development and execution.” (Barton, Lee, Resnick, 2019)
- Make sure we can accurately interpret and explain your machine learning model. Not only to translate the value and accuracy of your findings to executives, but help circumvent biased models. This book is a great place to learn how interpretability is a useful debugging tool for detecting bias in machine learning models.
- Reference a data science ethics checklist or framework.
Ultimately, we need to view technology as an enabler and not a savior. The impetus is on people and companies to practice and implement the principles of inclusion and diversity. Technology is a useful support system, but not something we should roll out as a “quick fix” to solve broader problems. But, it can educate us to make better choices, help us be consistent in our efforts, track our progress, and hold ourselves accountable to our mistakes.
Choosing ethical AI solutions for the enterprise
Ethical AI involves ensuring that AI systems and tools are designed, deployed, and maintained in ways that promote fairness, accountability, transparency, and social good, while minimizing harm, bias, and misuse. For companies, choosing AI-powered tools that align with their ethics is essential to maintaining trust, avoiding legal or social backlash, and promoting sustainable innovation.
Here are key principles and considerations for making AI ethical, along with how companies should approach the selection of AI tools:
Principle | Ethical AI | How companies should choose tools |
Fairness and Bias Mitigation | AI should be designed to avoid perpetuating bias and ensure fairness for all. | Assess if AI tools have mechanisms to identify, mitigate, and correct biases. Review training data and outcomes for fairness. |
Transparency and Explainability | AI decisions should be understandable and explainable to affected individuals. | Choose AI tools that offer explainability features and have clear documentation on how decisions are made. |
Accountability | AI systems should be accountable to humans, and responsible actors must be identifiable. | Ensure AI tools have oversight mechanisms and can be audited. Human oversight should be possible in critical decision-making processes. |
Privacy and Data Security | AI should protect individual privacy and handle data responsibly. | Select AI tools that comply with data protection laws (e.g., GDPR) and offer robust privacy and security features for data handling. |
Sustainability and Social Impact | AI should contribute to positive social outcomes and minimize harm. | Evaluate whether the AI tool aligns with the company’s sustainability goals and considers its broader social impact on communities. |
Compliance with Regulations | AI must adhere to legal and ethical guidelines set by governing bodies. | Ensure AI tools meet industry regulations, ethical standards, and are regularly updated to stay compliant with changing laws. |
Artificial intelligence ethics through inclusive language with Acrolinx
Inclusive language demonstrates awareness of the vast diversity of people in the world. Using inclusive language offers respect, safety, and belonging to all people, regardless of their personal characteristics.
Acrolinx supports your company at every stage of your diversity and inclusion journey — with inclusive language checking across all your company content. The Acrolinx inclusive language feature reviews your marketing, technical, support, and legal content for various aspects of inclusive language. It provides suggestions that make your content historically and culturally aware, gender neutral, and free from ableist language. Not to mention our inclusive language technology is also backed by an explainable, hand-crafted system to elevate transparency and reduce potential biases.
Want to learn more about how Acrolinx can help you roll out inclusive language across your organization? Make sure to download our latest eBook Can Technology Support Diversity, Equity, and Inclusion? Choosing the right solution for your enterprise D&I initiative.
Resources
Allen, James. A (2019) The Color of Algorithms: An Analysis and Proposed Research Agenda for Deterring Algorithmic Redlining, Fordham Urban Law Journal, Vol. 46. Retrieved from: https://ir.lawnet.fordham.edu/ulj/vol46/iss2/1
Frost, Stephen & Alidina, Raafi-Karim (2019) Building an Inclusive Organization: Leveraging the Power of a Diverse Workforce
Lee, Resnick, Barton (2019) Algorithmic bias detection and mitigation: Best practices and policies to reduce consumer harm. Retrieved from: https://www.brookings.edu/research/algorithmic-bias-detection-and-mitigation-best-practices-and-policies-to-reduce-consumer-harms/
Molnar, Christoph (2021) Interpretable Machine Learning: A Guide for Making Black Box Models Explainable, Retrieved from: https://christophm.github.io/interpretable-ml-book/
Are you ready to create more content faster?
Schedule a demo to see how content governance and AI guardrails will drastically improve content quality, compliance, and efficiency.
Kiana Minkie
She comes to her content career from a science background and a love of storytelling. Committed to the power of intentional communication to create social change, Kiana has published a plethora of B2B content on the importance of inclusive language in the workplace. Kiana, along with the Acrolinx Marketing Team, won a Silver Stevie Award at the 18th Annual International Business Awards® for Marketing Department of the Year. She also started the Acrolinx Diversity and Inclusion committee, and is a driving force behind employee-driven inclusion efforts.